The Shift Towards Local AI Processing
In recent years, the field of artificial intelligence (AI) has seen a remarkable transformation. As AI capabilities have expanded, so have the complexities and computational demands of the models that drive them. Traditionally, these models have relied heavily on cloud-based processing, leveraging vast server farms to handle the intensive computational load. However, this approach is increasingly showing its limitations, prompting a shift towards smaller, more efficient AI models that can be processed locally on devices.
The conventional reliance on large, complex AI models hosted in the cloud presents several challenges. Firstly, the computational costs are staggering. Running these models requires expensive hardware and considerable energy, a burden that is felt most acutely by smaller companies and startups that lack the resources of tech giants. Moreover, as the demand for AI services grows, so do the costs associated with maintaining and scaling cloud infrastructure. This financial strain is becoming unsustainable, driving the need for more cost-effective solutions.
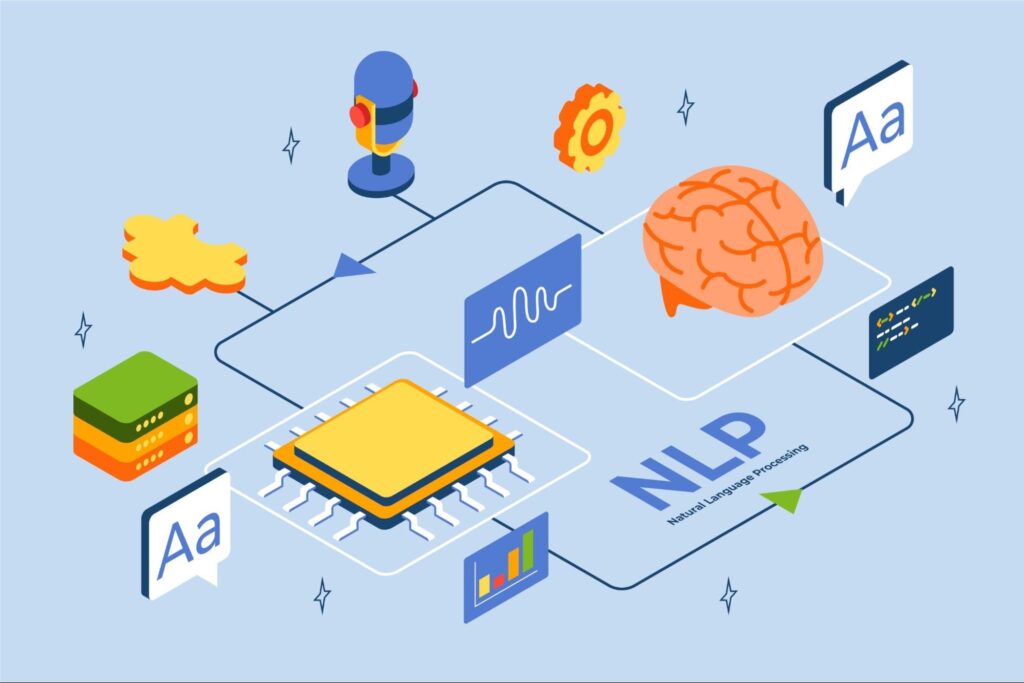
Addressing Privacy and Scalability Concerns
Privacy concerns are another significant issue. Processing sensitive data in the cloud raises the risk of data breaches and exposes personal information to potential misuse. For industries like healthcare and finance, where data privacy is paramount, this risk is particularly troubling. Local processing mitigates these concerns by keeping data on the device itself, ensuring that sensitive information remains secure and complies with stringent privacy regulations.
The dependency on cloud resources also poses scalability challenges. As more applications integrate AI functionalities, the resulting bottlenecks can lead to slower response times and decreased user satisfaction. This is especially problematic for real-time applications such as augmented reality (AR), virtual reality (VR), and autonomous driving, where even slight delays can significantly impact performance and user experience.
Local processing of AI models addresses these issues by bringing computation closer to the data source. By processing data locally, devices can offer enhanced privacy and security, reducing the risk of data breaches and ensuring compliance with privacy regulations. This approach also eliminates the need to transmit data to and from the cloud, resulting in faster response times and a more seamless user experience. Furthermore, reducing reliance on cloud infrastructure can significantly lower operational costs, making AI more accessible to smaller businesses and developers.
Technological Innovations Driving the Trend
Several technological advancements are driving the feasibility of smaller, efficient AI models. Model compression techniques such as pruning, quantization, and distillation help reduce the size of AI models without significantly compromising their performance. Quantization, for instance, reduces the precision of model parameters, decreasing memory usage and increasing processing speed. Techniques like Low Rank Adaptation (LoRA) streamline updates by freezing pre-trained model weights and injecting trainable layers.
The rise of edge computing is another critical factor. By allowing data to be processed on local devices or nearby servers, edge computing enhances performance by reducing latency and improving reliability. This decentralization is vital for applications requiring immediate data processing, such as industrial IoT and smart home devices.
Innovations in hardware are also playing a crucial role. Neuromorphic chips, which mimic the brain’s neural structure, offer substantial improvements in energy efficiency and computational power. These chips are designed to handle AI workloads more effectively than traditional processors, enabling sophisticated AI capabilities on devices with limited resources. This is particularly important for battery-operated devices like smartphones and wearables, where balancing performance with energy consumption is crucial.
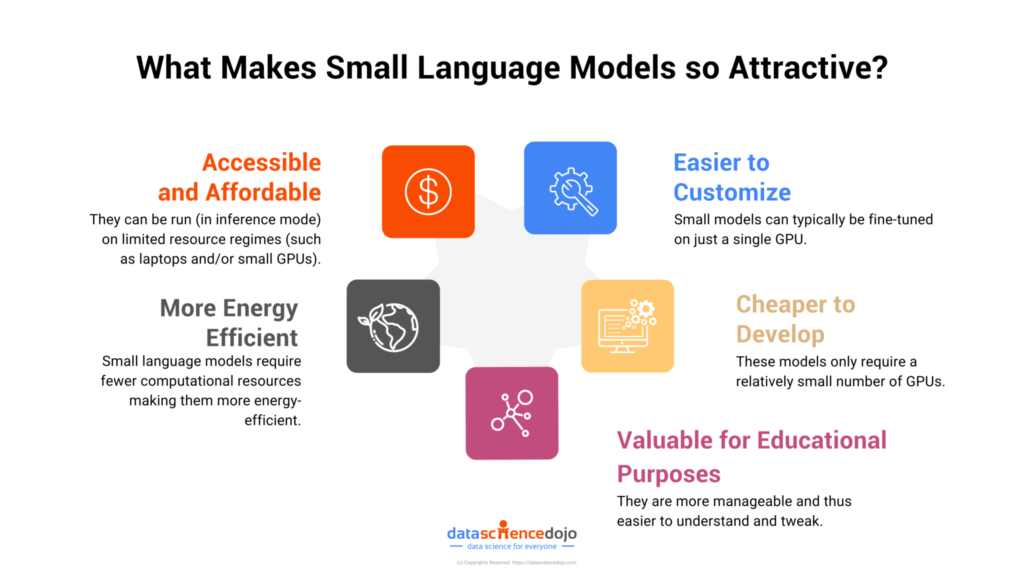
Real-World Applications and Future Outlook
The shift towards smaller AI models and local processing is already having a significant impact across various industries. In healthcare, AI-powered wearable devices can monitor health metrics in real-time, providing early warnings for potential health issues. Local processing ensures that personal health data remains secure while enabling timely interventions. This trend is driving a shift towards preventive healthcare and personalized health insights, empowering patients to take control of their health.
In the realm of consumer electronics, smart home devices such as voice assistants and security cameras benefit from faster response times and enhanced privacy by processing data locally. This not only improves user experience but also builds trust in AI products by ensuring that sensitive data is not shared unnecessarily. Autonomous vehicles, too, require rapid processing of vast amounts of data from sensors and cameras. Local AI processing reduces latency, improving the safety and reliability of these systems, which is crucial as the automotive industry moves towards fully autonomous driving.
Manufacturing and industrial IoT are other areas where local processing is making a difference. Real-time monitoring and control of industrial processes enhance efficiency and reduce downtime. AI models deployed on factory floors can monitor equipment health, predict failures, and optimize production schedules without relying on remote servers. This capability is transforming manufacturing operations, making them more resilient and responsive.
Challenges and Considerations
While the advantages of smaller AI models and local processing are clear, the transition is not without challenges. Local devices often have limited computational power and storage capacity compared to cloud servers. Developing efficient algorithms and hardware that can handle complex AI tasks within these constraints is a significant challenge. Moreover, while specialized hardware like neuromorphic chips can improve energy efficiency, running AI models locally still consumes power. Balancing performance with energy consumption remains a critical concern.
Software optimization is another hurdle. Developing software that can effectively leverage local hardware capabilities requires significant expertise in both AI and embedded systems. This includes optimizing models for different types of hardware and ensuring compatibility with existing software ecosystems. As technology continues to evolve, addressing these challenges will be essential to fully realize the potential of smaller AI models and local processing.
Future Prospects
As the demand for efficient and secure AI solutions grows, the trend towards smaller models and local processing is set to accelerate. This approach not only addresses current challenges but also opens up new possibilities for AI applications, driving innovation and transforming industries. By continuing to optimize AI models and leveraging advancements in hardware, we can achieve a future where AI is not only powerful but also accessible, secure, and sustainable.
The future of AI lies in balancing power with efficiency. Smaller AI models that can be processed locally are a significant step in this direction. They promise to democratize AI, making it accessible to a broader range of users and applications while addressing critical issues of privacy, cost, and scalability. As technology continues to evolve, we can expect to see even more sophisticated and efficient AI solutions that bring the benefits of artificial intelligence closer to everyone.
As AI continues to evolve, the focus on smaller, efficient models processed locally is poised to redefine the landscape. This shift not only addresses the pressing issues of privacy, cost, and scalability but also paves the way for a more inclusive and sustainable AI future. With ongoing advancements in model compression techniques, edge computing, and specialized hardware, the potential for locally processed AI is vast. The democratization of AI, driven by these innovations, promises to unlock new possibilities, making advanced AI capabilities accessible to all, from tech giants to small businesses and individual developers. The journey towards smaller AI models and local processing is just beginning, and its impact on the future of technology will be profound and far-reaching.
0 Comments